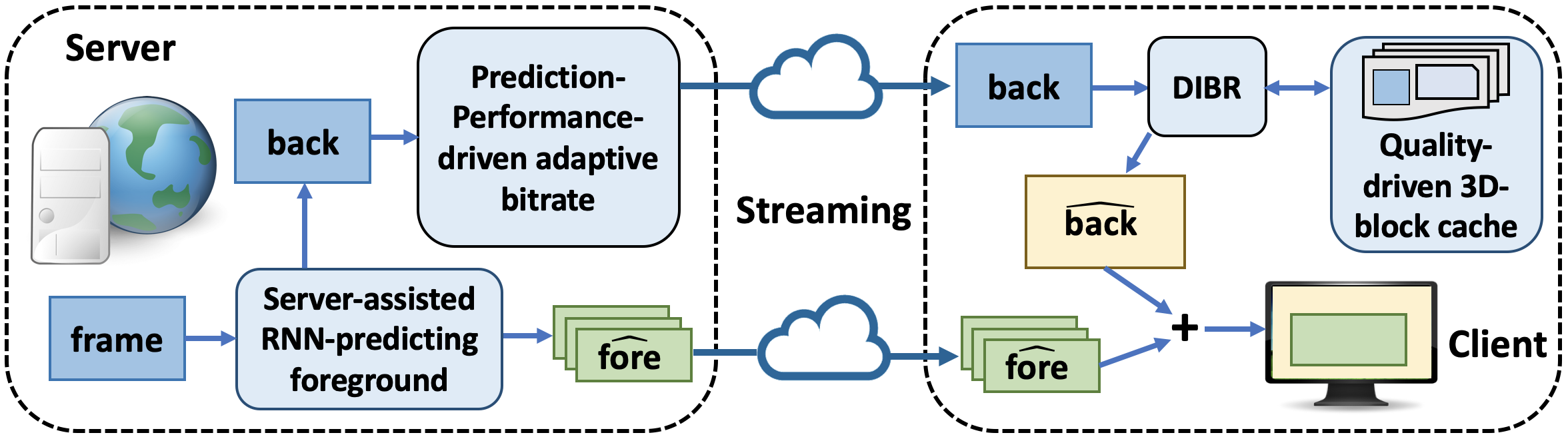
Thanks to the high-quality reconstruction and photorealistic rendering, NeRF (Neural Radiance Fields) has attracted extensive attention and achieved continuous improvement. Despite its impressive visual effect, the prohibitive reconstruction time limits its practical application in real life. Although significant acceleration has been achieved, there is still a considerable gap from real-time reconstruction, due to the need for tens of thousands of iterations. In this paper, a feasible solution thus is to reduce the number of required iterations by always training the underfitting rays samples. To this end, we propose an online active ray sampling strategy, ActRay. Since the states of rays are partially observable and outdated, a rendering-gradient-based loss propagation algorithm is presented to fully estimate the ray states. To balance exploration and exploitation, a UCB-based online reinforcement learning algorithm is proposed to adaptively select the most valuable and potential rays. We evaluate ActRay on both real-world and synthetic scenes, and the promising results show that it accelerates radiance fields training to 651% of the original speed. Besides, we test ActRay under all kinds of NeRF representations (implicit, explicit, and hybrid), demonstrating that it is general and effective to different representations. We believe this work will contribute to the practical application of radiance fields, because it has taken a step closer to real-time radiance fields reconstruction.
Table 1. The performance of Actray on Plenoxels
Quality (PSNR) | 28 | 30 | 31 | 32 | 33.5 |
Baseline (Iters) | 850 | 2400 | 4600 | 9700 | 15200 |
Ours (Iters) | 300 | 450 | 700 | 1700 | 3600 |
Acceleration | 283 % | 533 % | 651 % | 571 % | 422 % |
Table 2. The performance of Actray on Instant-NGP
Quality (PSNR) | 31 | 32 | 33 | 34 | 34.2 |
Baseline (Iters) | 1136 | 1888 | 3802 | 12368 | 18000 |
Ours (Iters) | 1024 | 1388 | 2307 | 6300 | 8609 |
Acceleration | 111 % | 135 % | 167 % | 196 % | 209 % |
Table 3. The performance of Actray on NeRF
Quality (PSNR) | 26 | 27 | 28 | 29 | 30 |
Baseline (Iters) | 9552 | 17641 | 34861 | 76757 | 223974 |
Ours (Iters) | 4620 | 5910 | 11250 | 22310 | 69400 |
Acceleration | 207 % | 298 % | 310 % | 344 % | 323 % |
BibTex
@inproceedings{10.1145/3610548.3618254, author = {Wu, Jiangkai and Liu, Liming and Tan, Yunpeng and Jia, Quanlu and Zhang, Haodan and Zhang, Xinggong}, title = {ActRay: Online Active Ray Sampling for Radiance Fields}, year = {2023}, booktitle = {SIGGRAPH Asia 2023 Conference Papers}, articleno = {97}, numpages = {10}, location = {Sydney, NSW, Australia}, series = {SA '23} }
ZGaming: Zero-Latency Cloud Gaming by Image Prediction
SIGCOMM'23